In today’s data-driven world, businesses or organizations rely heavily on data to make smarter decisions, optimize their processes for business growth, and of course gain a competitive edge. Behind the scenes, data engineers (professionals) play a crucial role in ensuring that raw data is collected, organized, and made flexible for analysis. As the organization gets heavily dependent on data, accordingly the demand for data engineers increases. This blog is your comprehensive guide on how to become a data engineer.
So, what exactly does it take to become a data engineer? Unlike data scientists, who analyze and interpret data, data engineers focus on designing, developing, and managing systems that handle massive amounts of data. From creating pipelines for real-time data processing to ensuring data security, this role is both highly technical and rewarding. In this blog we will be talking about several technical skills that helps individuals to cover entire data engineer roadmap
Well in the starting the journey to becoming a data engineer seems to be very difficult due to the wide variety of technical skills and tools involved to complete this journey. But now, with the structured approach any professional who is willing to switch their domain to data engineering can excel in this field.
In this blog we will be covering what data engineers do, the technical skills you need to become a successful data engineering professional, understanding how data engineering is different from data science and also covering the average data engineer salary.
If any student or working professional transitioned their careers in data engineering, or simply curious about this field, this blog will equip you with the knowledge and direction required to start on this exciting journey of how to become a data engineer in 2025 .
What is a Data Engineer ?
A data engineer is a highly skilled working professional who designs, builds, monitors and maintains computational systems and IT infrastructures to process and analyze large amount of data.
Data Engineer ensures and maintains data quality by collecting, storing and processing raw data into useful insights for data analysis. They create robust data pipelines and manage databases by extracting valuable insights from the organization’s raw data. This involves working with large and complex systems and implementing solutions to handle databases efficiently.
Tools used by data engineers are SQL, Airflow, AWS, Apache Spark, Hadoop, AWS, and Azure whereas tools used by data scientists are Python, R, Tensorflow, Visualization tools like Tableau or Power BI.
By building the foundation for data-driven decision-making, data engineers enable businesses to thrive in today’s competitive landscape. If you’re interested in becoming one, you’ll need a strong understanding of data architecture, programming, and big data technologies.
How To Become a Data Engineer ? Step-by-Step Guide
Data engineering is a dynamic and very vast field for managing and processing large-scale data. Here’s a detailed step-by-step guide to help you understand the entire roadmap for how to become a data engineer in 2025.
Understand the Role
Data engineers design, build, and maintain data pipelines, ensuring seamless integration, storage, and accessibility of data. They work with massive datasets to support analytics and machine learning models. Understanding their responsibilities and tools like Apache Kafka, Spark, and ETL processes is crucial.
Educational Qualifications
To become a data engineer, a bachelor’s degree in computer science, information technology & systems, data science is typically required. For those who are aiming for higher roles in the field of data engineering then they must go for the masters in the same.
Master Technical Skills
Programming Languages : Learn Java or Scala, Python for developing and managing data workflows.
Databases : Build familiarity with databases to cover knowledge in SQL for relationship databases, NoSQL databases like MongoDB and Cassandra.
Big Data Frameworks : Try to cover big data frameworks like Hadoop, Spark and Hive for handling massive datasets.
Cloud Computing : Learning cloud computing helps data engineers to manage data pipelines which includes AWS, Azure or Google Cloud.
Data Warehousing : Understand tools like Snowflake, Redshift or BigQuery for efficient data organization.
Gain Some Practical Experience
Once you have gained some practical knowledge then you should apply for internships or full time roles for practical exposure. You can also build your learning community or post your projects on platforms like GitHub, Kaggle .
Develop Soft Skills
Individuals should have problem solving capabilities, communication skills as well as teamwork for understanding and explaining technical solutions along with collaborating cross-functional teams.
Build Portfolio & Start Applying
Use professional platforms like GitHub or Kaggle to build your portfolio and connect different communities to share your work and build networks. Once you have done with all the basic buildings then start applying for junior roles for continuously upskilling yourself.
Stay updated to follow current technology and trends, participate in data engineering forums and follow industry trends to stay competitive.
What do data engineers do ?
Data engineers play a crucial role in managing and optimizing the flow of data within an organization. They are responsible for designing, building, and maintaining the systems and infrastructure that allow data to be collected, stored, and processed efficiently. Their main task is to create data pipelines, which are series of steps that move data from various sources—like databases, applications, or external APIs—into a centralized storage system, such as a data warehouse or data lake.
Once the data is in these systems, data engineers ensure it is clean, organized, and structured in a way that makes it easy for data analysts or data scientists to work with. This might involve tasks like removing duplicates, fixing errors, or transforming raw data into a more useful format. They use various tools and technologies like SQL, Python, Hadoop, and Spark to automate these processes and handle large volumes of data efficiently.
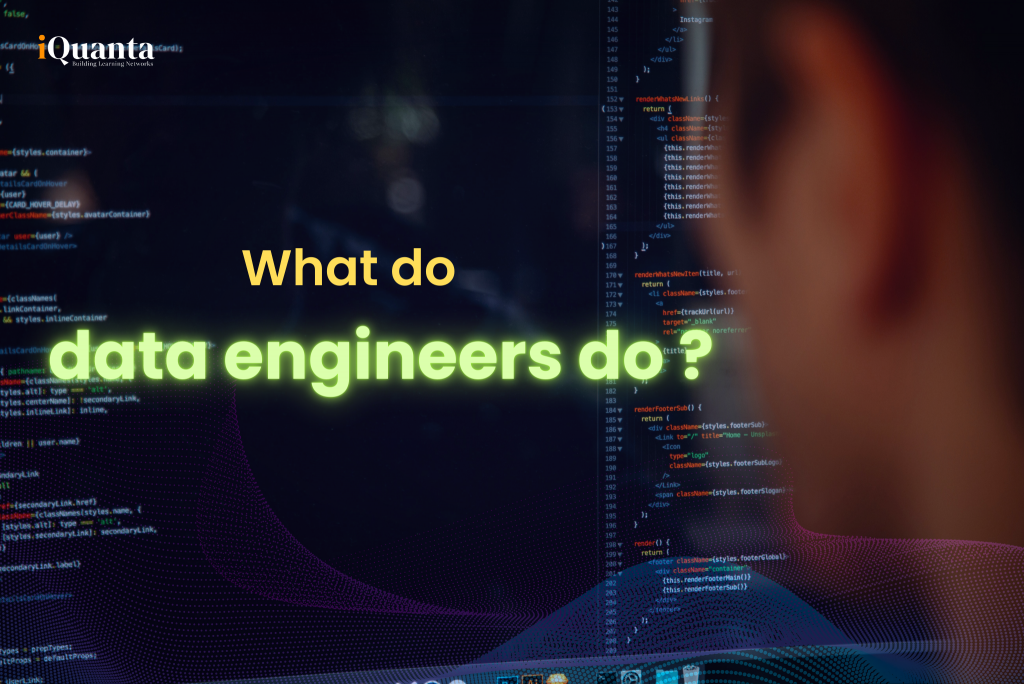
Ultimately, data engineers provide the foundation for data-driven decision-making within an organization. By ensuring data is accurate, reliable, and easy to access, they enable data scientists, analysts, and business teams to analyze the information and make insights that drive important business decisions. Without data engineers, companies would struggle to collect and process the vast amounts of data needed to stay competitive in today’s data-driven world.
Technical Skills Required To Become A Data Engineer
To become a data engineer in 2025 you need to cover these technical skills to ensures data engineer roadmap.
Programming Languages : Learn Java or Scala, Python for developing and managing data workflows.
Databases : Build familiarity with databases to cover knowledge in SQL for relationship databases, NoSQL databases like MongoDB and Cassandra.
Big Data Frameworks: Try to cover big data frameworks like Hadoop, Spark and Hive for handling massive datasets.
Cloud Computing : Learning cloud computing helps data engineers to manage data pipelines which includes AWS, Azure or Google Cloud.
Data Engineer Salary
Data Engineer Salary Breakdown (2025) in INR
Experience Level | INR Average Salary | Entry-Level (0-2 years) | Mid-Level (2-5 years) | Senior-Level (5+ years) | Lead/Principal |
Base Salary | ₹8,97,240 | ₹58,210 – ₹70,550 | ₹74,700 – ₹99,600 | ₹99,600 – ₹1,32,800 | ₹1,24,500 – ₹1,66,400 |
Data Engineer Salary by Region
Region | Average Salary | Entry-Level | Mid-Level | Senior-Level |
California (Bay Area) | ₹1,20,35,250 | ₹83,00,000 – ₹99,60,000 | ₹1,03,75,000 – ₹1,24,50,000 | ₹1,33,28,000 – ₹1,74,60,000 |
New York (NYC) | ₹1,04,37,500 | ₹74,70,000 – ₹91,50,000 | ₹91,50,000 – ₹1,16,50,000 | ₹1,24,50,000 – ₹1,49,00,000 |
Texas (Dallas, Austin) | ₹91,30,000 | ₹66,40,000 – ₹83,00,000 | ₹83,00,000 – ₹1,08,00,000 | ₹1,08,00,000 – ₹1,41,00,000 |
Florida (Miami) | ₹87,15,000 | ₹62,25,000 – ₹79,00,000 | ₹79,00,000 – ₹99,60,000 | ₹1,04,00,000 – ₹1,33,00,000 |
Midwest (Chicago) | ₹95,45,000 | ₹66,40,000 – ₹83,00,000 | ₹83,00,000 – ₹1,08,00,000 | ₹1,08,00,000 – ₹1,33,00,000 |
Washington D.C. Area | ₹1,08,10,000 | ₹79,00,000 – ₹95,50,000 | ₹95,50,000 – ₹1,20,50,000 | ₹1,24,50,000 – ₹1,49,00,000 |
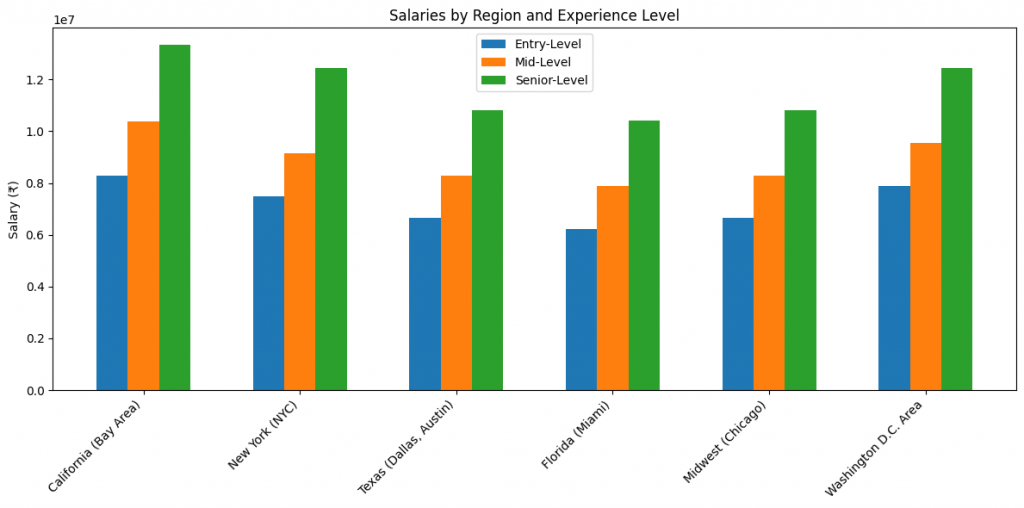
Data Engineer Salary by Industry
Industry | Average Salary | Entry-Level | Mid-Level | Senior-Level |
Technology/Software | ₹99,60,000 | ₹74,70,000 – ₹91,50,000 | ₹91,50,000 – ₹1,16,50,000 | ₹1,16,50,000 – ₹1,49,00,000 |
Finance/FinTech | ₹1,04,75,000 | ₹78,90,000 – ₹95,50,000 | ₹95,50,000 – ₹1,20,50,000 | ₹1,24,50,000 – ₹1,57,00,000 |
Healthcare/Pharma | ₹95,45,000 | ₹70,70,000 – ₹87,30,000 | ₹87,30,000 – ₹1,08,30,000 | ₹1,08,30,000 – ₹1,41,00,000 |
Retail/E-Commerce | ₹91,30,000 | ₹66,40,000 – ₹83,00,000 | ₹83,00,000 – ₹1,04,00,000 | ₹1,04,00,000 – ₹1,33,00,000 |
Government/Nonprofit | ₹83,00,000 | ₹58,30,000 – ₹74,70,000 | ₹74,70,000 – ₹95,50,000 | ₹99,60,000 – ₹1,24,50,000 |
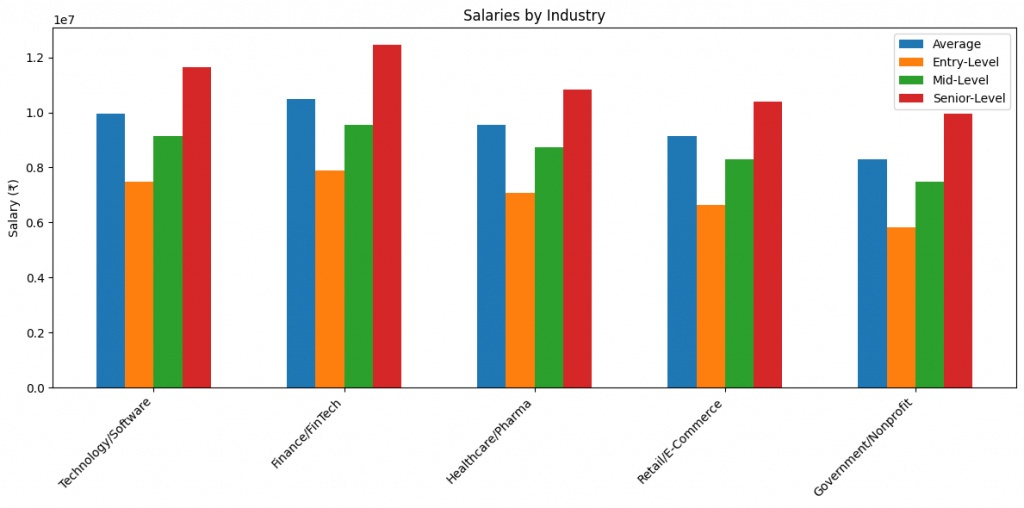
Factors Affecting Data Engineer Salary
- Experience: More years in the field or working with cutting-edge technologies typically leads to higher pay.
- Location: Salaries in tech hubs (like Bengaluru, Hyderabad, and Pune) tend to be higher due to the demand for skilled engineers.
- Education: A Master’s or PhD can increase salary expectations, though a strong skill set and experience often weigh more.
- Skills: Proficiency in cloud platforms (AWS, Azure), programming languages (Python, Scala), and big data technologies (Hadoop, Spark) can boost salary.
- Certifications: Certifications related to data engineering, cloud architecture, or specific technologies (like AWS or Google Cloud) can increase earning potential.
Frequently Asked Questions (FAQs)
How does a data engineer different from data scientists ?
Data Engineers are mainly responsible for building and maintaining the IT infrastructure which includes data storage, collection and processing whereas data scientists analyze, understand and interpret data workflow properly to predict trends and insights.
Tools used by data engineers are SQL, Airflow, AWS, Apache Spark, Hadoop, AWS, and Azure whereas tools used by data scientists are Python, R, Tensorflow, Visualization tools like Tableau or Power BI.
What is the typical workflow of data engineers ?
Here is the detailed overview of the typical workflow of data engineers:
- Understanding Requirements : Data engineers are dealing with stakeholders to define data needs.
- Collection of Data : Data engineers collect data from multiple sources such as websites, databases, APIs, as well as multiple cloud computing platforms.
- Data Cleaning : Clean and preprocess raw data for quality and consistency.
- Building Data Pipelines : Data engineers build data pipelines and develop ETL (Extract, Transform and Load ) processes for data workflow.
- Scalability : Data Engineer ensures that the platform needs to be scalable and well built to manage data efficiently.
- Monitor and Maintain : Data engineer’s responsibility is to ensure proper monitoring of pipelines and troubleshooting issues.
Is coding necessary to become a data engineer ?
Yes, coding is necessary for data engineering. Data engineers use Python, SQL, and sometimes Scala or Java to build ETL pipelines, manage databases, process data, and work with big data tools like Apache Spark and Hadoop. Proficiency in coding ensures efficient handling of data workflows and systems. You need to cover these basic technologies to ensure data engineer roadmap.
What coding languages are most commonly used by data engineers ?
The most commonly used programming languages by data engineers are Python ,Scala, Java as well as R.
What are some real-world applications of data engineering ?
There are some real world applications of data engineering includes :
- Entertainment : Streaming platforms like Netflix optimize their content recommendation system using processed data.
- Internet of Things : IoT technology is basically handling data from smart agents or devices to monitor real time analytics.
- Social Media : Supporting data-driven advertisements and organic user-engagement analytics.
- Healthcare : Healthcare industry is one of the great application of data engineering which process large scale patients data.